By: Sai Avinash Karri
What adjustments is Artificial Intelligence (AI) making to financial service providers' capital markets strategy? Financial service providers are always seeking ways to outperform rivals and get an advantage in the capital markets. Businesses are embracing more flexible business models that include AI and machine learning (ML) approaches in place of older technologies to achieve this.
Breaking Down Capital Markets
Investment portfolios for both individual and corporate clients are frequently managed by specialized capital market groups at financial institutions. All financial institutions must define best practices in performing market research, monitoring markets, detecting compliance gaps, and developing funding strategies, even though specific activities and assets vary across groups and businesses.
Markets are assumed to function normally when investors do their due diligence before making an investment decision. Earnings and losses are not exceptional nor unusual because prices represent the information that is readily available to investors. But what happens when automated systems support the transaction process for financial industry professionals? What happens when AI influences investment decisions rather than people? How has the relationship between financial institutions and their customers and capital suppliers changed as a result of AI-powered continuous risk modeling? Let's examine three ways that AI and automation are reshaping the capital markets.
Automation and Artificial Intelligence in Capital Markets Automating Business Processes
Robotic process automation (RPA) is a kind of automation software that takes over repetitive work from humans. RPA use cases are widespread in the front, middle, and back offices of capital market organizations. Firms employ RPA for updating client data in record systems, error reconciliation, and analyzing legal contracts in the middle and back office, which has historically been the area where RPA adoption has been most advantageous in practice. RPA gives financial companies a less expensive option, especially when the price of offshore middle-and back office processes grows.
RPA is used in the trading, investment research, and portfolio management divisions of the front office. Automated investor reports, for instance, can cut down on the time that financial professionals need to read, extract, and evaluate financial data. Imagine you're a trade analyst who needs to put together a daily report of OTC market activity. Without RPA, you'll probably need to manually gather and transmit data from different sources (your email, CRM database, online brokers, etc.). RPA makes use of bots to automatically update the data in the relevant database by reading and extracting structured data from spreadsheets and email attachments. An analyst can deliver internal and client updates in almost real-time by using bots to replace the labor-intensive procedure of copying and pasting information.
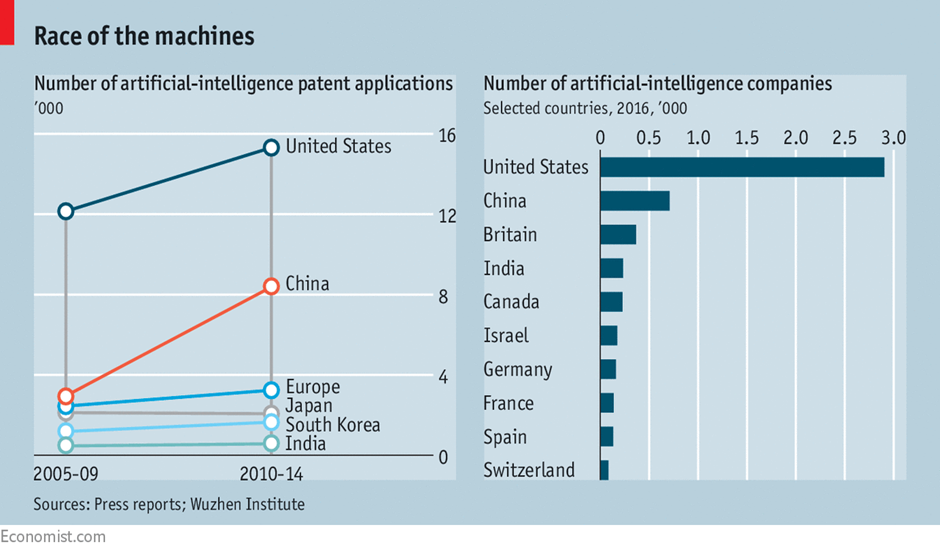
Investment companies are moving toward automating their activities using more sophisticated methods these days. However, a company's ability to scale RPA effectively inside an organization is a good sign that it is equipped to manage advanced automation.
Building Market Intelligence with Alternative Data
Financial institutions are employing AI to shift through unstructured data, a typically labor-intensive operation, which is not surprising given the growth of Big Data. Financial firms can create investment signals by using AI to manage large amounts of unstructured data sources.
AI bots can index websites, for instance, and alert financial professionals to pertinent statistics. Financial advisors can use data from social media posts and natural language processing (NLP) to measure consumer sentiment toward a given market.
Financial analysts who choose stocks using fundamental analysis may find the latter application useful. Financial organizations can utilize NLP to build predictive models for the future stock performance of companies based on history and current news about those companies. Current sales and trading trends, however, indicate that businesses are switching from using AI for predictive analysis (i.e., what is likely to happen) to prescriptive analysis (i.e. what should be done, based on likely outcomes).
Financial institutions are encouraged to use alternative data to learn things about their competitors' businesses that they might not learn from standard data. The majority of financial advisors recognized as "market leaders" use a variety of different data sources to provide alpha, control risk, and guide investment choices.
Improving Pre and Post-Trade Risk Analysis
Capital market AI is used by financial firms to better precisely track their risk exposure. In deploying advanced capital and risk-management solutions, financial sector organizations can benefit from AI and enhanced automation, according to a 2018 World Economic Forum report. The use of AI tools assists in the trade process:
Pre-trade risk analysis:
AI and advanced automation can help with adherence to the law, both now and in the future. For instance, financial institutions that have more than EUR/USD 50 billion in assets must exchange Initial Margin (IM) with their counterparties under phase 5 of the criteria for uncleared derivatives. More participants in the financial services sector will need to get ready for these changes because later stages of the regulation's implementation will also involve participants from the buy-side.
Financial organizations can improve the estimation of initial margins by utilizing AI/ML approaches (a form of collateral exchanged between parties to minimize risk exposure).
Continuous Risk Analysis:
With the introduction of continuous risk models, AI is also facilitating better compliance. Financial institutions can adjust their capital levels thanks to continuous risk models, which alert them in real-time to changes in risk exposure. Businesses can enhance compliance (e.g., change the amount of cash to have on hand) and boost revenue with AI-driven risk management technologies.
Post-Trade Risk Analysis:
It takes a lot of data sifting to find variations that lead to post-trade failures. Businesses can use AI/ML predictive modeling to find trends in incident data and predict upcoming incidents. Companies that can spot these trends can cut expenses and implement stronger incident management procedures because the post-trade process is more prone to errors in trading and operations.
By 2030, it's predicted that Japan's AI market would have increased from JPY 3.7 trillion in 2015. The largest AI sub-segment in 2015, valued at JPY 1.45 trillion, or nearly 39% of the total market value, was AI solutions for the wholesale and retail industries.
The Future of Capital Markets
Sector-wide, capital market companies are realizing greater benefits from integrating RPA into analysis and administrative tasks, AI trading technology, and predictive analytics systems. Improved capital efficiency and better risk management are two of these advantages, made possible by how quickly these technologies can produce insights into the health of a firm and its exposure to risk as well as complete duties like financial reporting.
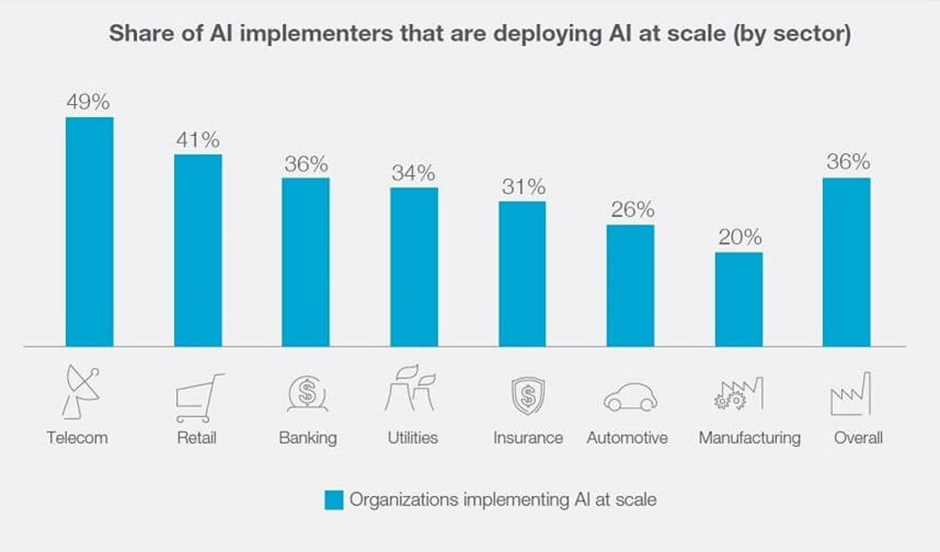
The advantages of exclusively employing AI to guide investment decisions tell a different tale. Many contend that AI systems cannot always outperform humans or "beat the market," especially when compared to professional investors.
The acceleration of AI is ultimately influenced by capital market firms in a similar way to how capital markets are impacted by AI. To ensure decision transparency with their clients and capital providers, enterprises need to maintain confidence in the quality of their data as more financial institutions embrace AI-aided investing methods.
Well explained!