- By Daksh Darji
The Capital market and the commodity market are two major financial pillars of global finance. Capital Market refers to a financial system where individuals, businesses, and government can issue and trade financial securities like stocks, bonds, and derivatives to raise long-term funds. In contrast, a system where commodities like oil, copper, lead, gold, and Aluminium are traded refers commodity market.
The relationship between the capital market and the commodity market is complex. But it is very crucial to comprehend some of the major reasons:
Commodities are used as raw materials for the production process. In case the price of raw materials increases there will be a direct impact on the increase of cost price for the firm leading to lower profit and declining stock price.
Commodities are usually used as a hedging material. Commodities prices typically increase along with inflation. This is due to the fact that commodities are frequently used as input, as a result, the prices frequently reflect production costs. Therefore, investors can use commodities to shield their portfolios from inflationary effects.
Portfolios can be diversified using commodities. Although commodities are frequently regarded as a risky asset class, they can also effectively diversify portfolios. Commodities can aid risk because they have a tendency to move in a different direction than stocks and bonds.
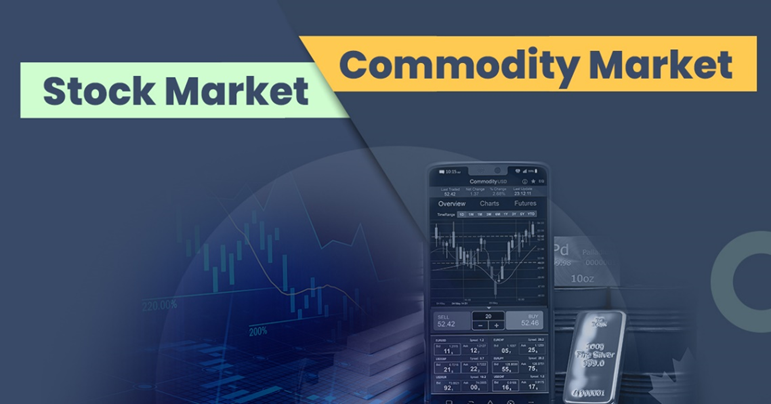
There are numerous ways to describe the intricate connection between capital markets and commodities. Here are a few examples of the various kinds of relationships:
· Direct Connection: Stock prices and commodity prices occasionally move in the same direction. For instance, higher transportation costs for businesses may result from rising oil prices, which may then cause lower profits and lower stock prices.
· Inverse Connection: In some cases, commodities may move in opposite to one another. For instance, when gold’s price increases, it may be perceived as a safe haven investment, causing investors to sell stocks and buy gold.
· Mixed Relationship: There is also a possibility of a mixed relationship between stocks and commodities. In case, a bad harvest may cause the price of wheat to increase, raising food producers' expenses and reducing their profits. On the other hand, it will also help the farmers and other businesses that produce or sell wheat.
CASE STUDY:
DYNAMIC RELATION BETWEEN COMMODITY MARKET AND CAPITAL MARKET –
The case study is based on two variables Crude oil from the commodity market and NSE Nifty from the capital market. The past six months of historical data are being collected and with the help of a data analysis test, it results in the relation between two variables. In this case study the dependent variable is Crude Oil and the independent variable is NSE Nifty.
GRANGER CASUALTY TEST
The Granger Causality test is a statistical test used to determine if one time series can predict another time series. In this case, Granger causality between two variables, "DCRUDEOIL" and "NIFTY," is being tested with a lag of 2.
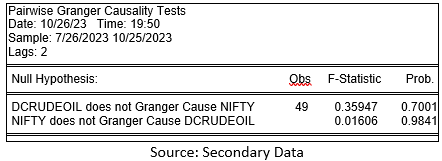
1. Null Hypothesis: "DCRUDEOIL does not Granger Cause NIFTY."
In this case, the null hypothesis is that "DCRUDEOIL does not Granger Cause NIFTY." The F-Statistic is 0.35947, and the probability associated with this test is 0.7001. Since the probability is relatively high (greater than the common significance level of 0.05), you do not have enough evidence to reject the null hypothesis. This suggests that, with a lag of 2, DCRUDEOIL does not Granger cause NIFTY.
2. Null Hypothesis: "NIFTY does not Granger Cause DCRUDEOIL."
Observed F-Statistic: 0.01606
Probability (Prob.): 0.9841
In this case, the null hypothesis is that "NIFTY does not Granger Cause DCRUDEOIL." The F-Statistic is 0.01606, and the probability associated with this test is 0.9841. Again, the high probability suggests that you do not have enough evidence to reject the null hypothesis. This means that, with a lag of 2, NIFTY does not Granger cause DCRUDEOIL.
In both cases, the null hypotheses are not rejected, which implies that there is no Granger causality between the two variables, DCRUDEOIL and NIFTY, within the specified lag of 2.
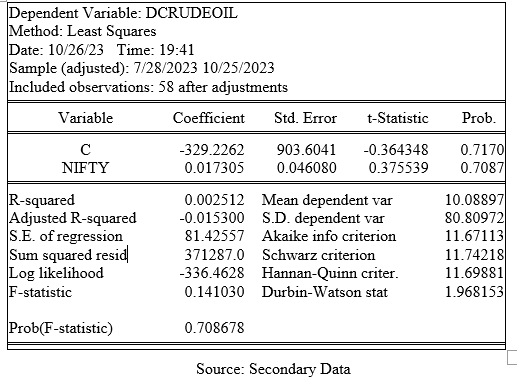
This means that changes in one of the variables do not predict or cause changes in the other variable based on the data and lag used in the test.
ORDINARY LEAST SQUARES
Ordinary Least Squares (OLS) is a statistical method used for estimating the parameters of a linear regression model. It is a fundamental technique in econometrics, statistics, and data analysis. In an OLS regression, the goal is to find the linear relationship between a dependent variable and one or more independent variables by minimizing the sum of the squared differences (residuals) between the observed and predicted values.
In our case though the Granger Causality test have shown no significant relationship between both the variables we have further conducted Ordinary least squares which have showcased that there exists a significant relationship between the
Dependent Variable – Crude Oil
Independent Variable- Nifty
Null Hypothesis- There is no significant impact of Nifty on Crude Oil
Alternative Hypothesis – There is a significant impact of Nifty on Crude oil
Coefficients:
Coefficient values represent the estimated impact of the independent variables on the dependent variables With a probability value of 0.7170 and with a coefficient value of NIFTY being 0.017305 suggests we to reject the null hypothesis and interpret that there exists a significant impact of the independent variable nifty on the dependent variable Crude oil
JOHANSEN CO-INTEGRATION TEST
Co-integration refers to a long-term equilibrium relationship between two or more variables. In the context of financial analysis, co-integration between variables suggests that they are interdependent and share a common long-term trend. Therefore, if some variables are co-integrated, they cannot be treated as independent variables, and their relationship must be accounted for in the analysis.
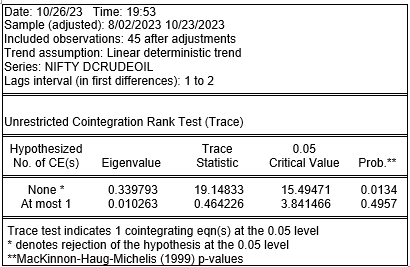
· For the hypothesis of "None," the test statistic (Trace) is 19.14833, and the critical value at the 0.05 significance level is 15.49471. The p-value (0.0134) is less than 0.05 (the 0.05 significance level), indicating that you should reject the null hypothesis of "None." This suggests that there is at least one cointegrating equation between the variables NIFTY and DCRUDEOIL.
· For the hypothesis of "At most 1," the test statistic is 0.464226, and the critical value at the 0.05 significance level is 3.841466. The p-value (0.4957) is greater than 0.05, indicating that you do not have enough evidence to reject the null hypothesis of "At most 1." This suggests that you cannot confirm that there is at most one cointegrating equation between the variables.
In summary, the cointegration test suggests that there is at least one cointegrating equation between the NIFTY and DCRUDEOIL series at the 0.05 significance level, as indicated by the rejection of the "None" hypothesis. However, it does not provide enough evidence to confirm the existence of at most one cointegrating equation. This implies that there is a long-term relationship between these two variables, but the exact nature of that relationship may involve more than one cointegrating equation.
Wald test
The Wald Test is a statistical test used to determine whether specific parameters or groups of parameters in a statistical model are jointly significant or equal to certain hypothesized values. It is commonly used in the context of linear regression, generalized linear models, and other statistical models. The primary purpose of the Wald Test is to assess the statistical significance and also interpret whether the relationship between the variables is short-term or long-term.
Null Hypothesis: There is a long-term relationship between the two variables
Alternative Hypothesis: There is a long-term relationship between the two variables
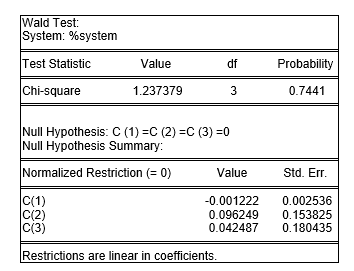
The result of the Wald Test indicates whether the null hypothesis is rejected or not. In this case, with the probability value of 0.7441 and the Chi-square statistic being at 1.23 which is not equal to zero it is inferred that the null hypothesis is rejected, and it suggests that the parameters or coefficients being tested are statistically different from the hypothesized values, and they have a significant effect on the model. There exists a long-term relationship between the two variables.
BOTTOM LINE AND FUTURE OUTLOOK
The present case examines the relationship between the commodity and capital markets using the past 3 months' data from 27th July 2023 to 25 October 26, 2023. Crude Oil price represents the commodity market and NSE Nifty is taken as the proxy for the capital market in India. The Granger causality test confirmed the presence of no directional causality which runs from Crude oil to stock price but Ordinary least squares confirmed that there is a significant impact of nifty fluctuations on the crude oil with a co-efficient value of 0.017 It is established from the Johansen cointegration test that gold price and stock price are cointegrated & VECM found the existence of a long-run equilibrium relationship between the two and the Wald test finally confirmed that there is a bi-directional relationship in the long -run between the two variables.
The Nifty and the price of crude oil can be significantly impacted by geopolitical concerns. This is due to the fact that geopolitical risks have the potential to cause supply disruptions & raise oil demand.
· Supply Disruptions
Oil prices may increase significantly if supply disruptions caused due to geopolitical tensions occur in major oil-producing regions like the Middle East. This might affect the nifty because it might cause India’s economy to grow more slowly and with a higher rate of inflation
· Growing Requirement for Oil
The demand for oil may rise as a result of geopolitical instability as businesses and investors look to protect themselves from rising energy costs. Moreover, this may lead to an increase in oil prices which would have a negative impact on the Nifty.
Comments